Our Robot
We specialize in building decentralized trading Robots for market predictions using Python, combining big data analytics, alternative data, AI & MS for optimized market performance. Our key focus is the efficiency of an automated crypto trading platform, enhancing your personal portfolio's effectiveness. We aim to help more people enjoy the benefits of this advanced trading solution.
RobotBulls News
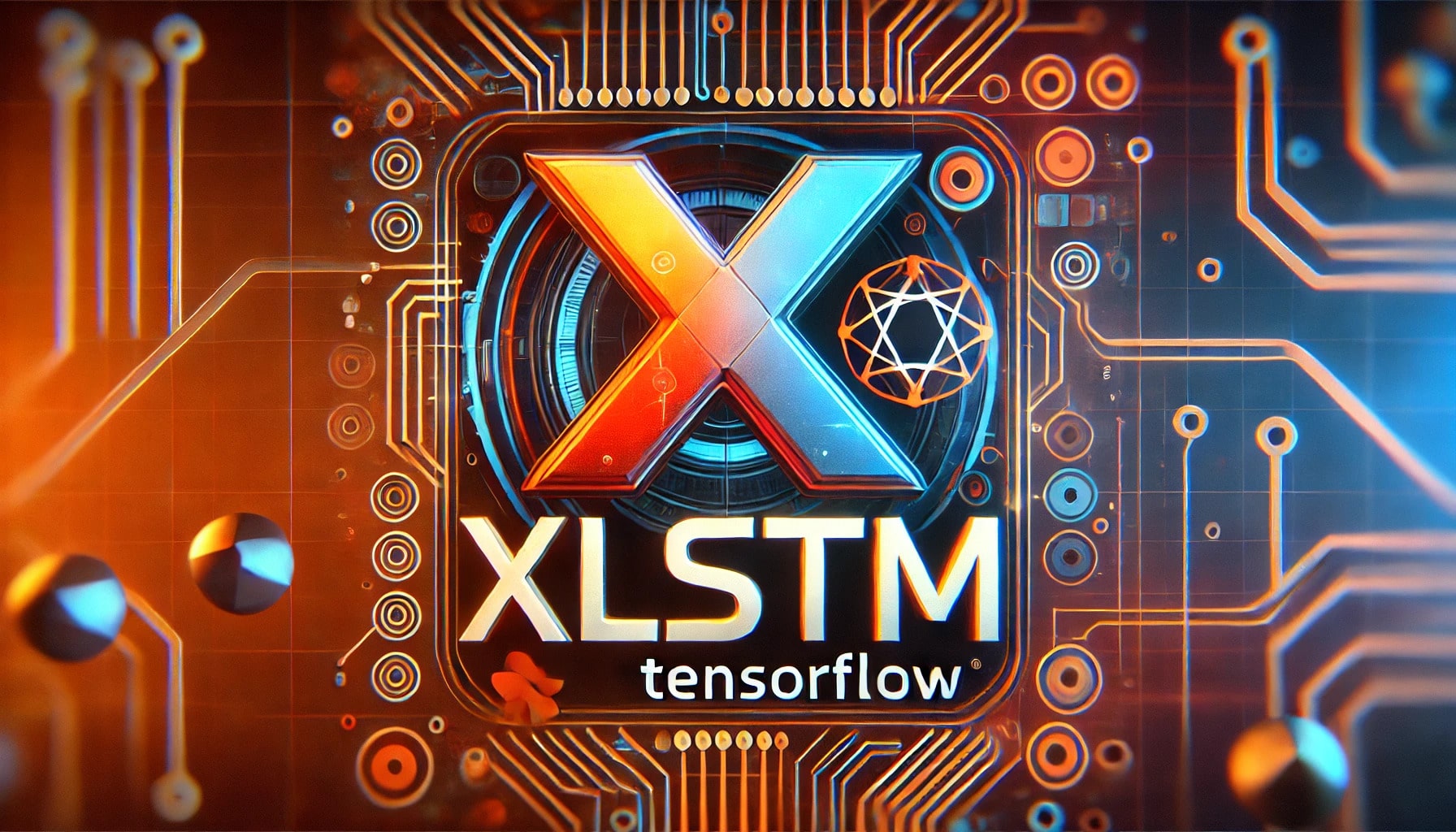
Time Series Forecasting Using TensorFlow and xLSTM
In today's data-driven world, predicting future trends based on historical data is crucial. To improve multivariate time series forecasting, we embarked on a project to develop an advanced xLSTM (Extended Long Short-Term Memory) model using TensorFlow...
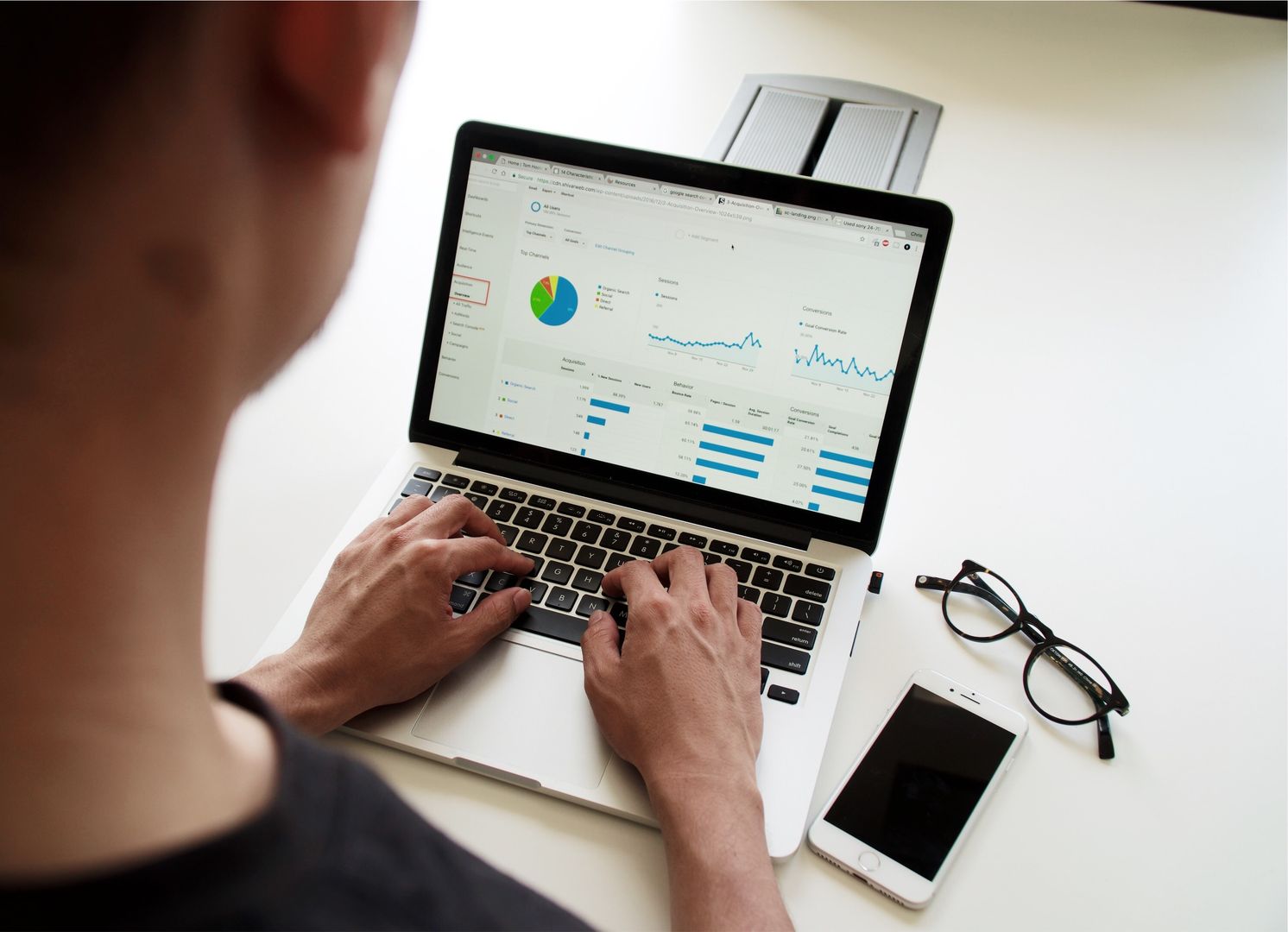
Democratizing Data Science: How Citizen Data Scientists are Transforming the Tech and Finance Industries in 2023
As the world continues to rapidly develop and become more data-driven, the need for data scientists has grown exponentially. However, not everyone has the technical...
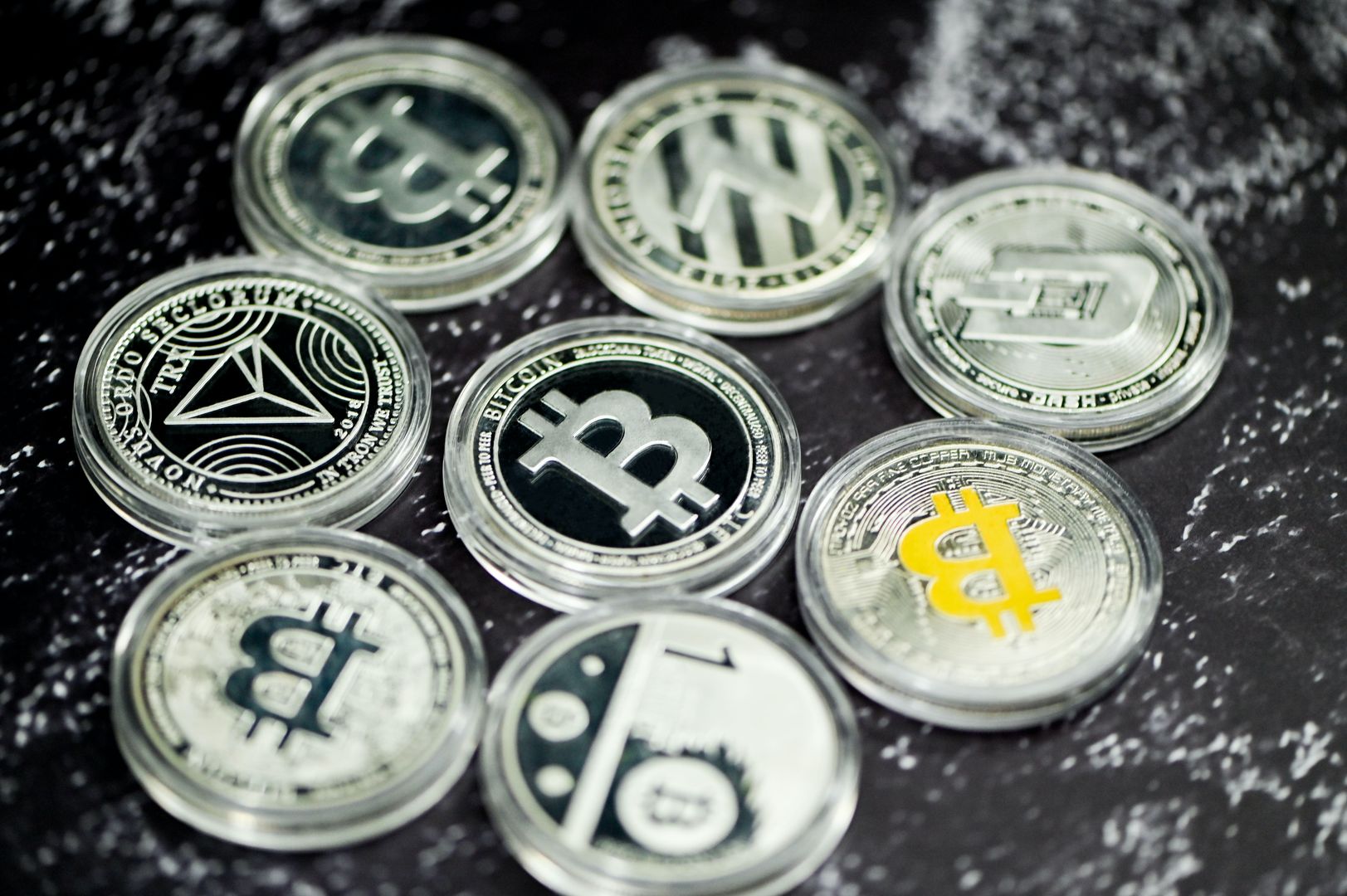
Embracing the Era of Embedded Finance: How API-Driven Innovations are Fueling the Convergence of Tech and Finance in 2023
In 2023, we are witnessing the rapid convergence of technology and finance, driven in large part by the rise of embedded finance and API-driven innovations....
RobotBulls has been aproved by the European Commission and Erasmus+ as an official internship partner and trusted company for professional development purposes.